Billing mistakes and claim denials cost the U.S. healthcare system 935 million per week and 48 billion annually (Source: Change Healthcare, 2023 via BusinessWire). Even minor errors, like typos or mismatched codes, can delay payments for months.
Claim scrubbing is the automated process medical billing clearinghouses use to detect and correct these mistakes before claims reach payers, safeguarding revenue and accelerating reimbursements. It generally reduces denials by up to 80-90%.
This guide explains:
- How claim scrubbing works,
- The key techniques clearinghouses rely on,
- How AI and automation are revolutionizing this critical billing safeguard.
Related Resource: Clearinghouse vs. Direct Billing: Which Claim Submission Method Fits Best?
What Is Claim Scrubbing in Medical Billing?
Claim scrubbing is an automated pre-submission review process that detects and corrects errors in medical claims before they reach insurance payers.
Purpose
- Identifies missing data (e.g., patient demographics, provider details).
- Flags coding errors (e.g., mismatched ICD-10/CPT codes, unbundling).
- Ensures formatting compliance with HIPAA and payer-specific rules.
Benefits
✅ Reduces denials by up to 80-90% (for preventable errors).
✅ Speeds up reimbursements by avoiding resubmissions.
✅ Cuts administrative costs by minimizing manual rework.
How Claim Scrubbing Works?
Clearinghouses and billing software use real-time validation rules (e.g., NPI checks, code edits, duplicate claim detection) to “scrub” claims. Advanced systems even use AI to predict denial risks.
Example: A claim missing a modifier (e.g., CPT 99213 without -25 for a separate E/M service) would be flagged and corrected before submission.
Why Do Clearinghouses Perform Claim Scrubbing?
Clearinghouses scrub claims to achieve critical operational goals:
1. Boost Clean Claim Rates
- Strive for clean claim rates exceeding 95%, minimizing rework. (Industry benchmark for optimal revenue flow)
- Prevent errors like missing modifiers, invalid codes, or mismatched diagnoses.
2. Ensure Compliance
- Meet HIPAA regulations for electronic claims (EDI standards).
- Adhere to payer-specific rules (e.g., Medicare’s NCD/LCD policies).
Related Resource: How Do Healthcare Clearinghouses Maintain HIPAA Compliance?
3. Reduce Administrative Costs
- Lower resubmission expenses (e.g., staff time, follow-up labor).
- Avoid appeals and avoidable A/R delays.
4. Protect Revenue Cycles
- Ensure faster and more predictable cash flows for providers.
- Reduce denial write-offs by catching errors in advance.
5. Enhance Payer Relationships
- Submit error-free claims to avoid payer audits or penalties.
- Maintain trust and smoother claim processing with insurers.
Failing to scrub claims results in:
❌ Costly denials (up to 30% of claims are initially denied for preventable errors).
❌ Delayed payments (average of 14–30 days added per resubmission).
❌ Compliance penalties (e.g., HIPAA fines for incorrect formatting).
Struggling with claim denials or delayed reimbursements?
Medibill RCM LLC, a trusted RCM medical billing company, specializes in:
✔ AI-Powered claim scrubbing to achieve 98%+ first-pass acceptance rates
✔ Seamless clearinghouse-EHR integration (Epic, Cerner, and niche PM systems)
✔ Full-service medical billing services with real-time denial tracking and predictive analytics
Get a free Revenue Cycle Assessment
Core Claim Scrubbing Techniques Used by Clearinghouses
Clearinghouses rely on multiple validation layers to thoroughly scrub claims:
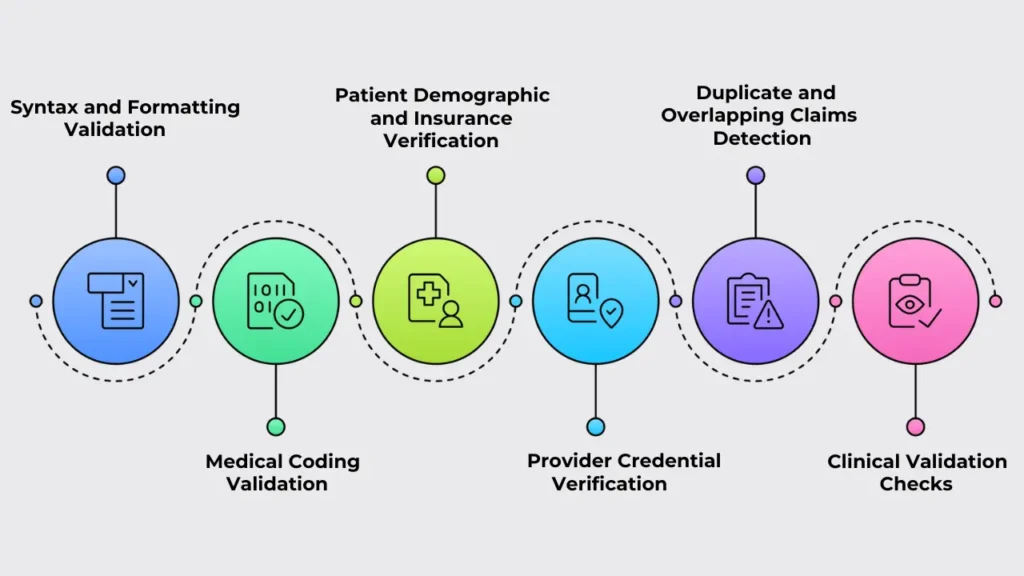
1. Syntax and Formatting Validation
- Detects missing required fields (e.g., patient ID, diagnosis codes, service dates).
- Identifies invalid characters (e.g., symbols in name fields) and non-compliant file structures.
- Validates compliance with ANSI X12 837 format and HIPAA transaction standards (e.g., 5010 requirements).
- Example: A claim missing the Place of Service (POS) code is flagged before submission.
Related Resource: Healthcare EDI: A Complete Guide to Electronic Data Interchange in Medical Billing
2. Medical Coding Validation
- Cross-checks ICD-10-CM, CPT®, and HCPCS Level II codes for accuracy.
- Verifies code linkage (e.g., CPT 99213 must align with a valid ICD-10 diagnosis).
- Flags invalid/retired codes (e.g., ICD-10-CM updates annually; outdated codes trigger rejections).
- Example: Billing CPT 93000 (EKG) without a supporting cardiac diagnosis (e.g., I20.9) prompts an error.
3. Patient Demographic and Insurance Verification
- Confirms active insurance coverage (via real-time eligibility checks).
- Validates patient DOB, policy numbers, and group IDs against payer databases.
- Detects coverage termination or coordination-of-benefits (COB) conflicts.
- Example: A claim with a policy number mismatch (e.g., transposed digits) is halted pre-submission.
4. Provider Credential Verification
- Validates NPI numbers and taxonomy codes (e.g., 207Q00000X for Family Medicine).
- Ensures providers are enrolled with the payer (e.g., Medicare PTAN checks).
- Prevents claims from non-participating or sanctioned providers.
- Example: A physical therapist’s claim submitted under a chiropractor’s NPI is rejected.
5. Duplicate and Overlapping Claims Detection
- Identifies duplicate claims (same service/date/patient).
- Flags overlapping dates (e.g., two surgeries billed on the same day).
- Cross-references prior submissions to prevent double billing.
- Example: Two claims for CPT 99214 on the same date for the same patient trigger an alert.
6. Clinical Validation Checks
- Confirms medical necessity per payer policies (e.g., LCD/NCD rules).
- Flags improbable age/gender combinations (e.g., prostate exam for a female patient).
- Detects unlikely service durations (e.g., 24-hour anesthesia for a 1-hour procedure).
- Example: Billing HCPCS L8600 (breast prosthesis) for a male patient raises a red flag.
Related Resource: How Medical Billing Clearinghouses Work?
Why These Techniques Matter?
- Prevent up to 90% of denials from common errors (e.g., coding, eligibility).
- Reduce A/R days by avoiding reprocessing delays (avg. 14–30 days per resubmission).
- Ensure compliance with CMS, HIPAA, and commercial payer rules.
How Clearinghouses Implement Claim Scrubbing?
Clearinghouses implement claim scrubbing through a multi-step process:
Step | Action | Purpose |
---|---|---|
1 | Data Ingestion | Converts all incoming claims into standardized formats (ANSI X12 837) |
2 | Rule Engine Execution | Applies automated edits for coding, compliance, and payer rules |
3 | Error Flagging | Identifies and categorizes issues (critical vs. warnings) |
4 | Provider Feedback | Delivers clear error reports with correction guidance |
5 | Claim Correction | Allows providers to fix and resubmit claims quickly |
Key Benefits:
✅ Prevents up to 80-90% of denials from common errors
✅ Speeds up payments by submitting clean claims upfront
✅ Reduces manual rework with automated error detection
Technologies Powering Advanced Claim Scrubbing
1. NLP (Natural Language Processing) Engines
Purpose: Extracting and validating clinical terms from unstructured text (e.g., physician notes, claims) is a well-documented use case for NLP in Healthcare.
Example Vendors:
- Amazon Comprehend Medical is a widely used NLP service for healthcare text analysis.
- Linguamatics (now part of IQVIA) specializes in clinical NLP for data mining.
2. Machine Learning (ML) Models
Purpose: ML models analyze historical claims data to predict denials, detect anomalies, and improve accuracy.
Example Vendors:
- Olive AI (though it shut down in 2023, it was a key player in AI-driven claims automation).
- Change Healthcare (now part of Optum) offers predictive analytics for claims processing.
3. Robotic Process Automation (RPA)
Purpose: Automating repetitive tasks (e.g., data entry, claim validation) to reduce manual errors.
Example Vendors:
- UiPath and Automation Anywhere are leading RPA tools in healthcare revenue cycle management.
Related Resource: Top 10 Clearinghouses in Medical Billing: Best Picks for Fast Claims & Fewer Denials
Examples of Errors Commonly Detected During Claim Scrubbing
Claim scrubbing detects a wide range of errors, including:
- ICD-10 Coding Mismatches: Example: Using a pediatric code for an adult patient.
- Missing Authorization Numbers: Example: Surgical procedures lacking pre-authorization IDs.
- Gender-Specific Procedure Code Conflicts: Example: Billing a prostate exam for a female patient.
- Patient Policy ID Mismatches: Example: Invalid or expired policy numbers tied to patients.
Each error type can directly lead to a claim denial or delayed payment.
Common Challenges in Claim Scrubbing
While claim scrubbing is critical for revenue flow, clearinghouses and providers face constant hurdles:
1. Frequent Payer Policy Updates)
Problem: Payers update rules monthly (e.g., Medicare Local Coverage Determinations, commercial payer edits).
Impact: Without real-time updates, scrubbing tools flag “errors” based on outdated rules or miss new requirements.
Solution: Automated systems integrate with payer bulletins and FHIR APIs to sync changes instantly.
2. Specialty-Specific Coding Complexities
Examples:
- Cardiology: Stress test supervision (93015) vs. global billing, modifier -26 for the professional component.
- Urology: Prostate biopsy coding (55700), post-op global periods with modifier -24.
- OBGYN: Global maternity care (59400) vs. split-pregnancy billing.
- Internal Medicine: Chronic care management (99490) overlapping with AWV (Annual Wellness Visit).
- Pediatrics: Well-child visits (99391) vs. sick visits (99213).
Impact: Generic scrubbing rules may wrongly reject valid claims or miss specialty-specific errors.
Solution: Specialty-focused claim-scrubbing templates and provider education loops.
3. False Positives vs. Missed Errors
The Tightrope: Overly aggressive scrubbing flags valid claims (e.g., legitimate modifier 59 use), while lax rules miss real errors.
Data: ~15–20% of scrubbed claims require manual review due to false positives.
Fix: AI-driven predictive analytics learn from provider-specific billing patterns to reduce noise.
4. Legacy System Limitations
Outdated Tech: Older clearinghouse software struggles with NLP-based note review or real-time eligibility checks.
Workaround: Hybrid human-AI audits for high-risk claims (e.g., surgeries, costly infusions).
Audit your denial reasons quarterly. If more than 10% of scrubbed claims are denied for “payer policy” errors, your scrubbing tool may need updates.
Future of Claim Scrubbing: Trends for 2025 and Beyond
Claim scrubbing continues to evolve rapidly:
- Predictive AI Models: Systems will predict and fix claims even before submission.
- Blockchain Validation: Smart contracts will certify claim authenticity in real-time.
- Self-Learning Scrubbers: AI systems will adapt scrub rules dynamically based on new payer data.
Industry analysts project that by 2026, most claim validations will incorporate autonomous AI assistance to improve efficiency and accuracy.
FAQ: Claim Scrubbing in Medical Billing
1. What is the main goal of claim scrubbing?
Claim scrubbing identifies and corrects errors before submission to reduce denials and accelerate reimbursements.
2. What errors does claim scrubbing detect?
It catches syntax errors, coding mismatches, missing authorizations, duplicate claims, and patient demographic inaccuracies.
3. How does AI enhance claim scrubbing?
AI analyzes historical denial patterns to predict and flag errors in real-time, improving accuracy dynamically.
4. Does claim scrubbing ensure 100% clean claims?
Advanced scrubbing tools can achieve >97% clean claim rates, drastically reducing rework.
5. What’s the difference between syntax and clinical validation?
- Syntax validation: Ensures correct formatting (e.g., field lengths, required data).
- Clinical validation: Verifies medical necessity (e.g., code compatibility, authorization rules).
6. Why is real-time scrubbing critical?
Immediate error correction prevents claim rejections, avoiding 15–30-day delays in revenue cycles.
7. Which technologies power modern claim scrubbing?
Top tools include NLP, machine learning, RPA, and rule-based engines for automated error detection.